Profile
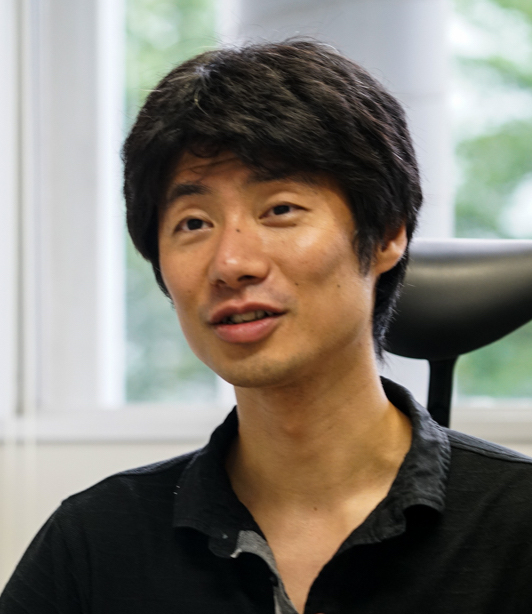
Adjunct Professor, Department of Mathematical Informatics, Graduate School of Information
Science and Technology, University of Tokyo
RIKEN Center for Brain Science, Team Leader
2-1 Hirosawa, Wako-shi, Saitama 351-0198
Tel: +81-48-467-9644
Fax: +81-48-467-9670
E-mail:taro.toyoizumi@riken.jp
Curriculum Vitae
Mar. 2001 | Graduated from the Department of Physics, School of Science, Tokyo Institute of Technology |
---|---|
Mar. 2003 | Graduated from the Master Course of the Department of Complexity Science and Engineering, Graduate School of Frontier Sciences, The University of Tokyo |
Mar. 2006 | Graduated from the Doctor Course of the Department of Complexity Science and Engineering, Graduate School of Frontier Sciences, The University of Tokyo |
Apr. 2006 – Feb. 2008 | Japan Society for the Promotion of Science Postdoctoral Fellow (RIKEN Brain Science Institute, Center for Theoretical Neuroscience, Columbia University) |
Mar. 2008 – Feb. 2010 | The Robert Leet and Clara Guthrie Patterson Trust Postdoctoral Fellow (Center for Theoretical Neuroscience, Columbia University) |
Apr. 2010 – Mar. 2011 | Special Postdoctoral Researcher, RIKEN Brain Science Institute |
Apr. 2011 – Mar. 2018 | Team Leader, RIKEN Brain Science Institute |
Apr. 2018 | Team Leader, RIKEN Center for Brain Science |
Apr. 2019 | Adjunct Professor, Department of Mathematical Informatics, Graduate School of Information Science and Technology, University of Tokyo |
Research Themes
Computational Neuroscience, Theory of Neural Adaptation using
Statistical Physics and Information Theory Tools
Our research is in the field of Computational Neuroscience. Computer
models are used to study how information is processed in the brain and
how the brain circuits adapt to and learn from the environment. We
employ analytical techniques from statistical physics and information
theory to investigate key functional properties for neuronal circuits.
We use these techniques to reduce diverse experimental findings into a
few core concepts that robustly explain the phenomena of interest.
We are particularly interested in activity-dependent forms of
plasticity in the brain, which are known to have large impacts on
learning, memory, and development. With the aid of mathematical
models, we seek a theory that unites the cellular level plasticity
rules and the circuit level adaptation in different brain areas and
animal species. Efficacy of neurons to represent and retain
information is estimated from the structure and behavior of resulting
circuits.
Selected Publications
-
Isomura T and Toyoizumi T.: “Error-Gated Hebbian Rule: A Local
Learning Rule for Principal and Independent Component Analysis”
Scientific Reports , 8, 1835 (2018), doi:10.1038/s41598-018-20082-0 -
Buckley C L and Toyoizumi T.: “A theory of how active behavior
stabilizes neural activity: neural gain modulation by closed-loop
environmental feedback”
PLOS Computational Biology , 14, e1005926 (2018), doi:
10.1371/journal.pcbi.1005926 -
Kuśmierz Ł and Toyoizumi T.: “Emergence of Lévy walks from
second-order stochastic optimization”
Physical Review Letters, 119, 250601 (2017), doi: 10.1103/PhysRevLett.119.250601 -
Tajima S, Mita T, Bakkum D, Takahashi H, and and Toyoizumi T.:
“Locally embedded presages of global network bursts”
Proc. Natl. Acad. Sci, 114, 9517-9522 (2017), doi: 10.1073/pnas.1705981114 -
Huang H and Toyoizumi T.: “Clustering of neural code words revealed by
a first-order phase transition”
Physical Review E, 93, 062416 (2016), doi: 10.1103/PhysRevE.93.062416 -
Shimazaki H, Sadeghi K, Ishikawa T, Ikegaya Y, and Toyoizumi T.:
“Simultaneous silence organizes structured higher-order interactions
in neural populations.”
Sci Rep, 5, 9821 (2015), doi: 10.1038/srep09821 -
Toyoizumi T, Kaneko M, Stryker MP, and Miller KD.: “Modeling the
dynamic interaction of Hebbian and homeostatic plasticity”
Neuron, 84(2), 497-510 (2014), doi: 10.1016/j.neuron.2014.09.036 -
Toyoizumi T, Miyamoto H, Yazaki-Sugiyama Y, Atapour N, Hensch TK, and
Miller KD.: “A theory of the transition to critical period plasticity:
inhibition selectively suppresses spontaneous activity” Neuron, 80(1),
51-63 (2013), doi: 10.1016/j.neuron.2013.07.022 -
Toyoizumi T and Abbott LF.: “Beyond the edge of chaos: Amplification
and temporal integration by recurrent networks in the chaotic regime”
Physical Review, E 84(5), 051908 (2011), doi: 10.1103/PhysRevE.84.051908 -
Toyoizumi T, Aihara K, and Amari S.: “Fisher information for
spike-based population decoding.”
Phys Rev Lett, 97(9), 98102 (2006), doi: 10.1103/PhysRevLett.97.098102 -
Toyoizumi T, Pfister JP, Aihara K, and Gerstner W.: “Generalized
Bienenstock-Cooper-Munro rule for spiking neurons that maximizes
information transmission.”
Proc Natl Acad Sci U S A, 102(14), 5239-44 (2005), doi: 10.1073/pnas.0500495102