|
|||||
|
|
Year: 2018
Hiroshi KORI
Profile
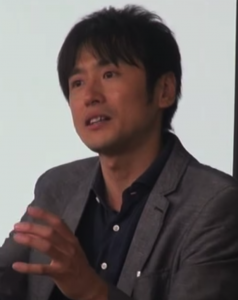
Graduate School of Frontier Sciences
The University of Tokyo
Professor
5-1-5 Kashiwanoha, Kashiwa-shi, Chiba-ken 277-8561
Tel: +81 4 7136 3936
E-mail:kori@k.u-tokyo.ac.jp
Biography
March 2003 | Doctor of Physics, Department of Physics, Graduate School of Science, Kyoto University |
---|---|
April 2004 | Research Fellow of Max Planck Society (Fritz Haber Institute |
March 2005 | Alexander von Humboldt Research Fellow (Fritz Haber Institute) |
April 2006 |
Research Fellow, Department of Mathematics, Hokkaido University |
March 2008 | Assistant Professor, Ochadai Academic Production, Ochanomizu University |
April 2012 | Associate Professor, Affiliation: Department of Computer Sciences, Ochanomizu University |
September 2018 | Professor, Graduate School of Frontier Sciences, The University of Tokyo |
Research Topics
I am working on various dynamical systems, such as
– Synchronization
– Complex networks
– Biological rhythms, circadian rhythms, jet lag
– Locomotion
– Power grids, transportation networks
– Self-organization, pattern formation
– Chemical reactions
– Micro-Macro links in nonlinear non-equilibrium systems
Selected Publications
-
- H. Kori, Y. Yamaguchi, H. Okamura: “Accelerating recovery from jet lag: prediction from a multi-oscillator model and its experimental confirmation in model animals”, Scientific Reports 7, 17466 (2017)
- H. Kori, Y. Kuramoto, S. Jain, I.Z. Kiss, J.L. Hudson: “Clustering in Globally Coupled Oscillators Near a Hopf Bifurcation: Theory and Experiments”, Phys. Rev. E 89, 062906 (2014)
- Y. Yamaguchi, H. Kori, H. Okamura et al: “Mice Genetically Deficient in Vasopressin V1a and V1b Receptors Are Resistant to Jet Lag”, Science 342, 85 (2013)
- I. Imayoshi, H. Kori, R. Kageyama et al.: “Oscillatory Control of Factors Determining Multipotency and Fate in Mouse Neural Progenitors”, Science 342, 1203 (2013)
- I.Z. Kiss, C.G. Rusin, H. Kori, J.L. Hudson: “Engineering Complex Dynamical Structures: Sequential Patterns and Desynchronization”, Science 316, 1886 (2007)
Mathematical Informatics of Social Complex Systems
|
|||||
|
We explore the universal properties underlying large-scale social
|
seisanken – omi
Profile

Associate ProfessorInstitute of Industrial Science, The University of Tokyo Department of Mathematical Informatics, Graduate School of Information Science and Technology, The University of Tokyo
Aihara lab., Institute of Industrial Science, The University of Tokyo, 4-6-1 Komaba, Meguro-ku, Tokyo 153-8505, Japan.
Tel: +81-3-5452-6697 (Ext. 56697)
Fax:
E-mail:omi@sat.t.u-tokyo.ac.jp
Curriculum Vitae
March 2007 | Bachelor of Science from Faculty of Science, Kyoto University |
---|---|
March 2009 | Master of Science from Department of Physics, Graduate School of Science, Kyoto University |
March 2012 | Ph.D. in Science from Department of Physics, Graduate School of Science, Kyoto University |
April 2012 | Researcher, Japan Science and Technology |
April 2013 | Japan Society for the Promotion of Science Fellowship for Young Scientists |
April 2016 | Project Research Associate, Institute of Industrial Science, The University of Tokyo |
April 2018 | Project Associate Professor, Institute of Industrial Science, The University of Tokyo |
Research Themes
Our main topics is time-series analysis. We especially focus on the statistical analysis of point process data, which describe events that occur irregularly in time. Our research includes
(1) the development of estimation and forecast method based on Bayesian statistics and
(2) its application to earthquake, economic, and social data.
Selected Publications
- T. Omi, Y. Hirata, and K. Aihara,
“Hawkes process model with a time-dependent background rate and its application to high-frequency financial data”,
Physical Review E 96, 012303 (2017). - T. Omi, Y. Ogata, Y. Hirata, and K. Aihara,
“Forecasting large aftershocks within one day after the main shock”,
Scientific Reports 3, 2218 (2013). - T. Omi and S. Shinomoto,
“Optimizing time histograms for non-Poissonian spike trains”,
Neural Computation 23, 3125 (2011).
Yoshihiro Kanno
教員紹介

Department of Mathematical Informatics, Graduate School of Information Science and Technology, University of Tokyo Professor
7-3-1 Hongo, Bunkyo-ku, Tokyo, 113-8656 Eng. 6 Bldg. Room 435
Tel: +81-3-5841-6913 (ext. 26913)
Fax:+81-3-5841-6886
E-mail: kanno@mist.i.u-tokyo.ac.jp
Education and Employment
March 1998 | B. Eng., Kyoto University |
---|---|
March 2000 | M. Eng., Kyoto University |
September 2002 | Dr. Eng., Kyoto University |
March 2004 | Assistant Professor, Department of Urban and Environmental Engineering, Kyoto University |
May 2006 | Assistant Professor, Department of Mathematical Informatics, The University of Tokyo |
September 2008 | Associate Professor, Department of Mathematical Informatics, The University of Tokyo |
April 2015 | Associate Professor, Materials and Structures Laboratory, Tokyo Institute of Technology |
April 2016 | Associate Professor, Laboratory for Future Interdisciplinary Research of Science and Technology, Tokyo Institute of Technology |
October 2017 | Professor, Mathematics and Informatics Center, The University of Tokyo |
Research Interests
Modeling and algorithms of mathematical optimization and their applications to applied mechanics and structural design
- Continuous optimization and applied mechanics: convex optimization, complementarity, duality and their applications to structural optimization, contact mechanics, plasticity, etc.
- Robust optimization and its applications: Optimization with uncertain data, robust optimization of structures, robustness evaluation of uncertain systems, etc.
Selected Publications
- Y. Kanno, “A fast first-order optimization approach to elastoplastic analysis of skeletal structures,” Optimization and Engineering, 17, 861–896 (2016).
- Y. Kanno, “Nonsmooth Mechanics and Convex Optimization,” CRC Press, Boca Raton (2011).
- Y. Kanno, J. A. C. Martins, A. Pinto da Costa, “Three-dimensional quasi-static frictional contact by using second-order cone linear complementarity problem,” International Journal for Numerical Methods in Engineering, 65, 62–83 (2006).
Mathematical Programming Laboratory
|
|||
|
|
Collaborative Research Center for Innovative Mathematical Modeling
|
||||
|
|
Professor Gouhei TANAKA
Faculty Staff Information

Project Associate Professor
International Research Center for Neurointelligence
Department of Mathematical Informatics, Graduate School of Information Science and Technology
Department of Electrical Engineering and Information Systems, Graduate School of Engineering
Room N308, IRCN, Faculty of Medicine Bldg. 1, The University of Tokyo, 3-7-1 Bunkyo-ku, Hongo, Tokyo 113-0033, Japan.
Phone: not available
E-mail:gtanaka@g.ecc.u-tokyo.ac.jp
[Website]
Research theme
-
Brain-like energy-efficient information processing
For realizing next-generation information processing systems, it is indispensable to miniaturize devices and make structures compact for enhancement of energy efficiency. We aim to develop mathematical methodologies for making brain-like computing systems energy efficient such that efficient computing is realized with low power and high speed.
-
Applications of machine learning and advanced mathematical methods
Machine learning technologies have enabled to efficiently perform tasks that have been manually handled by people. We aim to mathematically formulate problems in fields that are not approached by machine learning and mathematical modeling, and solve the problems by combining appropriate machine learning methods and advanced mathematical techniques.
-
Mathematical studies on medical and social systems
It is becoming possible to obtain real data on medical and social systems due to the developments of sensor devices and measurement techniques. We aim to propose effective control strategies for solving medically and socially important problems and improving quality of life.
-
Network robustness
Networked systems are ubiquitous in the world, such as the Internet, power networks, and biological networks. Networking often accompanies a risk that a partial failure causes a breakdown of the whole system. We are investigating how network robustness depends on network structure, dynamics, and element interactions. Our aim is to develop a design method of robust networks and a recovery method of damaged networks.
Recent Publications
-
-
G. Tanaka, R. Nakane, T. Takeuchi, T. Yamane, D. Nakano, Y. Katayama, and A. Hirose
Spatially Arranged Sparse Recurrent Neural Networks for Energy Efficient Associative Memory
IEEE Transactions on Neural Networks and Learning Systems, vol. 31, issue 1, pp. 24-38 (2020). DOI: 10.1109/TNNLS.2019.2899344A. Matsuki and G. Tanaka
Intervention threshold for epidemic control in susceptible-infected-recovered metapopulation models
Physical Review E, vol. 100, 022302 (2019).
G. Tanaka et al.
Recent Advances in Physical Reservoir Computing: A Review
Neural Networks, vol. 115, pp. 100-123 (2019).Z. Tong and G. Tanaka
Hybrid pooling for enhancement of generalization ability in deep convolutional neural networks
Neurocomputing, vol. 333. pp. 76-85 (2019).G. Tanaka, E. Dominguez-Huttinger, P. Christodoulides, K. Aihara, and R. J Tanaka
Bifurcation analysis of a mathematical model of atopic dermatitis to determine patient-specific effects of treatments on dynamic phenotypes
Journal of Theoretical Biology, vol. 448, pp. 66-79 (2018). -
System #1 lab.
|
|||
|
|
Ayumu Matani
Person

Associate Professor
Department of Information Physics and Computing
Graduate School of Information Science and Technology
7-3-1 Hongo, Bunkyo-ku, Tokyo 113-8656
Tel: 03-5841-7768
Fax:
E-mail:matani@isp.ac
[web page]
Career
1991 | M.Eng, Graduate School of Scientific Engineering, Osaka University |
---|---|
1991 | Researcher, Osaka Gas Co. Ltd. |
1995 | Assistant, Graduate School of Information Technology, Nara Institute of Science and Technology |
1998 | Ph. D, Graduate School of Scientific Engineering, Osaka University |
1998 | Assistant Professor, Graduate School of Engineering, the University of Tokyo |
1999 | Associate Professor, Graduate School of Frontier Sciences, the University of Tokyo |
2012 | Associate Professor, Graduate School of Information Science and Technology, the University of Tokyo |
Research Projects
We study cognitive neuroengineering, the engineering background is signal processing, instrumentation, information and communication, and electric and electronic circuit.
In electroencephalogram (EEG) recording associated with cognitive science experiments, the independent variables of the EEG are time, space (EEG channel), and epoch (or trial). In analyses of the EEG, a variety of temporal and spatial filters have been proposed so far and they play intrinsic and exclusive roles with respect to each other. If any epoch filter were created, it would provide a special role that both temporal and spatial filters are not able to play. For instance, we proposed epoch filters in order to analyze cross frequency coupling (2,3).
In the electrophysiological mechanism of neurons, the post-synaptic potentials generate dendritic currents, the dendritic currents flow out from neurons as a distributed current in the head after producing membrane potentials, and return to the original neurons. When the spatial sum of the dendritic current is measured as a voltage drop on the scalp, the measuemnt will be EEG. If an impedance is attached on the scalp, it modulates a portion of the dendritic currents and thereby would indirectly have an effect on the membrane potentials that originate the portion. For instance, we successfully shortened reaction time of a visual selective response task (1).
Publications
- 1) A. Matani, M. Nakayama, M. Watanabe, Y. Furuyama, A. Hotta, and S. Hoshino, Transcranial extracellular impedance control (tEIC) modulates behavioral performances, PLoS ONE, e0102834, 2014.
- 2) A. Matani, Y. Naruse, Y. Terazono, N. Fujimaki, and T. Murata, Phase-Interpolated Averaging for Analyzing Electroencephalography and Magnetoencephalography Epochs, IEEE Trans. on Biomedical Engineering, vol. 58, no. 1, pp. 71-80, 2011.
- 3) A. Matani, Y. Naruse, Y. Terazono, T. Iwasaki, N. Fujimaki, and T. Murata, Phase-Compensated Averaging for Analyzing Electroencephalography and Magnetoencephalography Epochs, IEEE Trans. on Biomedical Engineering, vol. 57, no. 5, pp. 1117-1123, 2010.